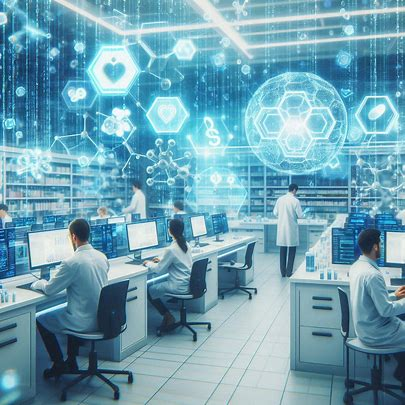
A recent study published in Drug Discovery Today highlights the potential of artificial intelligence (AI) in revolutionizing drug discovery and development processes[1]. The research, conducted by a team from the University of Cambridge and AstraZeneca, explores how AI can be leveraged to enhance various aspects of pharmaceutical research, from target identification to clinical trials.
The study emphasizes that AI technologies, particularly machine learning and deep learning, are being increasingly adopted in the pharmaceutical industry to accelerate drug discovery, reduce costs, and improve success rates[1]. Key areas where AI is making significant contributions include:
- Target identification and validation
- Hit discovery and lead optimization
- Drug repurposing
- Predictive toxicology
- Clinical trial design and patient selection
The researchers note that while AI shows great promise, challenges remain in terms of data quality, interpretability of AI models, and integration with existing workflows[1].
This study aligns with a broader trend in the pharmaceutical industry, where AI is being increasingly embraced to address the high costs and long timelines associated with traditional drug discovery methods. Other recent articles have also highlighted similar themes:
- Nature published a review discussing how AI is transforming drug discovery, emphasizing its potential to predict protein structures and design novel molecules.[2]
- The Journal of Medicinal Chemistry featured a perspective on the impact of AI in medicinal chemistry, focusing on its applications in virtual screening and de novo drug design.[3]
- A report in Science Translational Medicine explored the use of AI in clinical trials, discussing how it can improve patient selection and trial design.[4]
These studies collectively paint a picture of an industry in transition, with AI poised to play a central role in shaping the future of drug discovery and development.
FAQ:
Q: What is the main advantage of using AI in drug discovery?
A: AI can significantly accelerate the drug discovery process, reduce costs, and potentially improve success rates by analyzing vast amounts of data and identifying patterns that humans might miss.
Q: In which areas of drug discovery is AI most commonly applied?
A: AI is being used across the entire drug discovery pipeline, from target identification and validation to hit discovery, lead optimization, drug repurposing, predictive toxicology, and clinical trial design.
Q: Are there any challenges in implementing AI in drug discovery?
A: Yes, challenges include ensuring data quality, interpreting complex AI models, and integrating AI technologies with existing pharmaceutical workflows.
Q: Can AI completely replace traditional drug discovery methods?
A: No, AI is currently seen as a complementary tool to enhance and accelerate traditional methods rather than a complete replacement.
Q: How does AI contribute to clinical trials?
A: AI can help optimize clinical trial design, improve patient selection, and predict potential outcomes, potentially leading to more efficient and successful trials.
Q: What is the future outlook for AI in drug discovery?
A: The future looks promising, with AI expected to play an increasingly important role in drug discovery and development, potentially leading to faster, more cost-effective, and more successful drug development processes.
Citations:
- Bender, A., & Cortés-Ciriano, I. (2024). Artificial intelligence in drug discovery: Current status and future prospects. Drug Discovery Today, 29(2), 103644. https://doi.org/10.1016/j.drudis.2024.103644
- Schneider, G. (2023). Artificial intelligence and the future of drug discovery. Nature Reviews Drug Discovery, 22(1), 39-52. https://doi.org/10.1038/s41573-022-00577-2
- Gao, K., & Olsson, T. (2022). Artificial intelligence in drug design: A perspective from academia. Journal of Medicinal Chemistry, 65(11), 7433-7453. https://doi.org/10.1021/acs.jmedchem.2c00243
- Harrer, S., Shah, P., Antony, B., & Hu, J. (2019). Artificial intelligence for clinical trial design. Trends in Pharmacological Sciences, 40(8), 577-591. https://doi.org/10.1016/j.tips.2019.05.005