Introduction
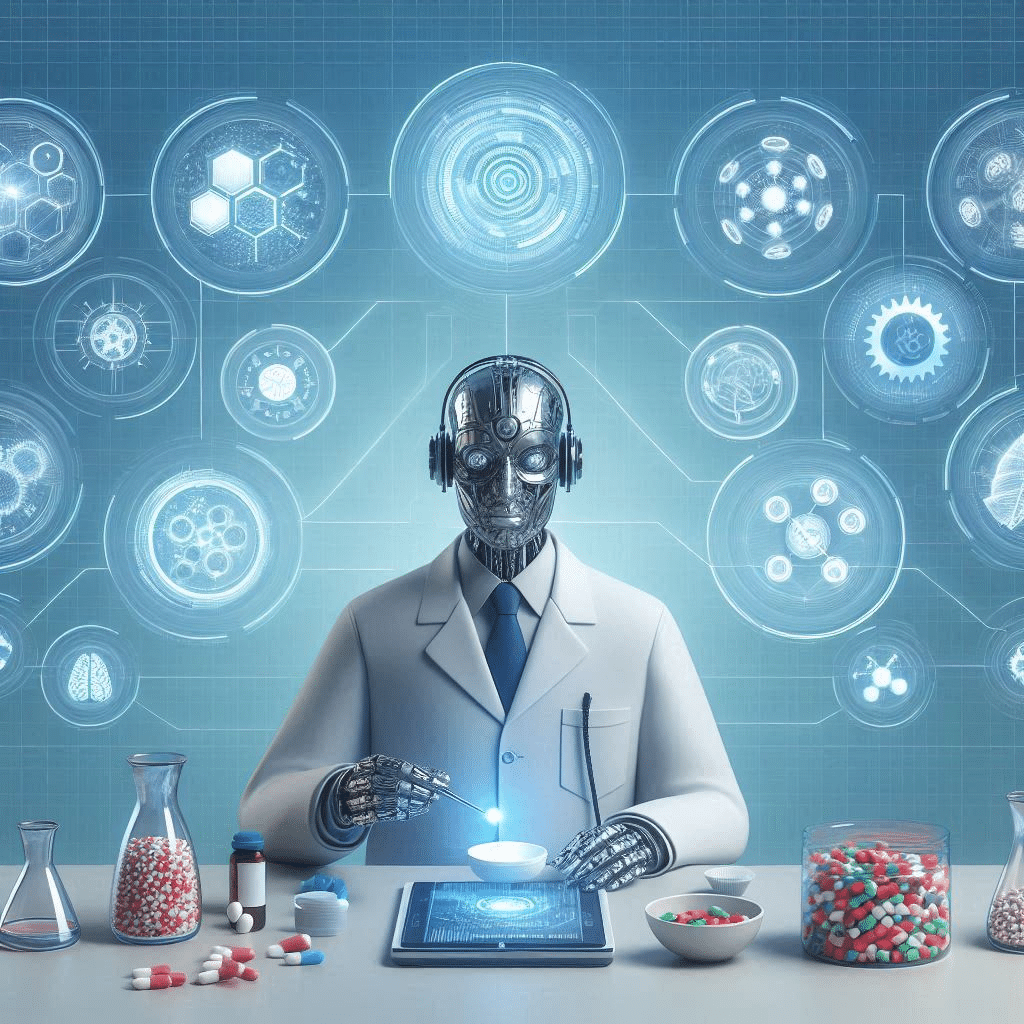
The pharmaceutical industry is undergoing a significant transformation with the advent of machine learning methods. These methods have the potential to revolutionize the drug discovery process by enhancing the efficiency and accuracy of identifying and developing new drug candidates. This article will explore how machine learning can be leveraged to optimize generic drug development, focusing on the key applications and challenges in this area.
Applications of Machine Learning in Generic Drug Development
- Finding Drug Biosimilars
- Predictive Analytics and Natural Language Processing: Generic drug companies can use predictive analytics and natural language processing to search through databases of brand-name drug compounds to find similar compounds that can be used to make generic drugs. This involves preprocessing pharmaceutical data, labeling it according to various properties such as solubility and crystal structures, and then running it through machine learning algorithms to identify correlations and potential biosimilars.
- Researching Drug Compound Crystal Structures
- Predictive Analytics: Predictive analytics can be used to determine how the shapes of compounds will react to certain methods of manufacturing and other drug development processes. This involves training algorithms on data connecting the names of drug molecules to their crystalline shapes, which can help generics companies determine the crystal structures of their biosimilars and ensure structural integrity.
- Salt and Polymorph Screening
- Machine Learning: Machine learning can be used to determine the solubility of a compound, ensuring it maintains its effectiveness over time. This involves analyzing large databases of past drugs, molecules, and research related to past clinical trials to form predictions about the solubility of biosimilars.
Challenges and Opportunities
- Access to Training Data
- Partnerships and Data Availability: One of the significant challenges generic drug companies face is accessing useful training data for machine learning models. This can be overcome by forming partnerships with healthcare companies or other organizations that have relevant data.
- Efficiency and Speed
- Automated Workflows: Machine learning can significantly speed up the drug discovery process by automating workflows and reducing the need for manual intervention. For example, researchers at Carnegie Mellon University developed an efficient automated workflow that identified compounds with high binding affinity to the target protein among thousands of congeneric ligands, outperforming traditional expert medicinal chemist-guided campaigns.
- Unbiased Search
- Agnostic Approach: Machine learning approaches can provide an unbiased search for optimal drug candidates, unencumbered by historical biases in chemical space. This can lead to the discovery of novel scaffolds and compounds that might have been overlooked by traditional methods.
Conclusion
Machine learning has the potential to significantly optimize generic drug development by enhancing efficiency, accuracy, and the discovery of novel compounds. By leveraging predictive analytics, natural language processing, and machine learning algorithms, generic drug companies can streamline their research and development processes, leading to faster and more effective drug discovery.
References
- Filella-Merce, I. (2023). Optimizing Drug Design by Merging Generative AI With Active Learning Frameworks. arXiv preprint arXiv:2305.06334.
- Carnegie Mellon University. (2023). Researchers Develop Active Learning Workflow to Optimize Drug Design. Retrieved from https://www.cmu.edu/chemistry/news/2023/0630_gutkin-gusev-journal.html
- Machine learning applications in drug development. (n.d.). Retrieved from https://www.sciencedirect.com/science/article/am/pii/S2001037019303988
- Rashid, M. (2020). Artificial intelligence in drug discovery and development. PMC – NCBI. Retrieved from https://www.ncbi.nlm.nih.gov/pmc/articles/PMC7577280/
- Emerj. (2019). Artificial Intelligence for Generic Drug Companies. Retrieved from https://emerj.com/ai-sector-overviews/artificial-intelligence-generic-drug-companies/