Table of Contents
I. Introduction
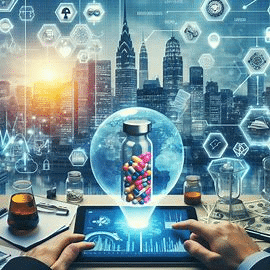
In the ever-evolving landscape of pharmaceutical research and development, a revolutionary force is reshaping the way we approach drug discovery and repurposing. This force is none other than Artificial Intelligence (AI) and Machine Learning (ML). As we stand on the cusp of a new era in healthcare, these technologies are proving to be game-changers, offering unprecedented opportunities to accelerate drug development, reduce costs, and ultimately, improve patient outcomes.
Drug repurposing, the process of finding new uses for existing drugs, has long been a strategy employed by researchers to expedite the drug development process. However, traditional methods of drug repurposing are often time-consuming and resource-intensive. Enter AI and ML – these cutting-edge technologies are transforming the field, enabling researchers to analyze vast amounts of data, identify patterns, and make predictions with remarkable speed and accuracy.
In this comprehensive exploration, we’ll delve into the fascinating world of AI and ML in drug repurposing. We’ll uncover how these technologies are revolutionizing the pharmaceutical industry, examine their applications, and discuss the challenges and opportunities that lie ahead. So, fasten your seatbelts as we embark on this thrilling journey into the future of drug discovery!
II. Understanding Drug Repurposing
A. Definition and Importance
Drug repurposing, also known as drug repositioning or drug reprofiling, is the process of identifying new therapeutic uses for existing drugs that are already approved for other indications. This approach has gained significant traction in recent years due to its potential to dramatically reduce the time and cost associated with bringing new treatments to market.
“Drug repurposing represents a significant innovative option for therapies for rare diseases. Indeed, among the over 7,000 identified rare diseases affecting 4% of the worldwide population, less than 6% of them have an approved treatment option.”[4]
The importance of drug repurposing cannot be overstated. Traditional drug development is a lengthy and expensive process, often taking 10-15 years and costing billions of dollars to bring a single new drug to market. In contrast, repurposed drugs have already undergone safety testing and have established pharmacokinetic profiles, potentially shaving years off the development timeline and significantly reducing costs.
Moreover, drug repurposing offers a lifeline for patients with rare or neglected diseases, where the economic incentives for developing new drugs are often lacking. By finding new uses for existing drugs, researchers can potentially provide treatments for these underserved populations more quickly and cost-effectively.
B. Traditional Approaches
Historically, drug repurposing has relied on serendipitous discoveries or hypothesis-driven approaches. Researchers would observe unexpected beneficial effects of a drug in clinical trials or practice, or they would use their knowledge of disease mechanisms to hypothesize potential new uses for existing drugs.
While these methods have led to some notable successes – such as the repurposing of sildenafil (Viagra) from a heart medication to a treatment for erectile dysfunction – they are inherently limited by human cognitive capacity and the ability to process vast amounts of biomedical data.
Traditional approaches to drug repurposing often involve:
- Literature-based discovery: Researchers manually comb through scientific literature to identify potential connections between drugs and diseases.
- Phenotypic screening: Existing drugs are tested in cellular or animal models of different diseases to identify potential therapeutic effects.
- Target-based screening: Drugs are screened against known disease targets to identify potential new indications.
- Pathway or network analysis: Researchers analyze biological pathways and networks to identify drugs that might affect disease-related processes.
While these methods have yielded results, they are time-consuming, labor-intensive, and often limited in scope. This is where AI and ML enter the picture, offering the potential to revolutionize the drug repurposing process.
III. The Emergence of AI and ML in Drug Discovery
A. Brief Overview of AI and ML
Before we dive into the specifics of how AI and ML are transforming drug repurposing, let’s take a moment to understand these technologies.
Artificial Intelligence (AI) refers to the simulation of human intelligence in machines that are programmed to think and learn like humans. It encompasses a wide range of capabilities, from problem-solving and planning to learning and perception.
Machine Learning (ML), a subset of AI, focuses on the development of algorithms and statistical models that enable computer systems to improve their performance on a specific task through experience. In other words, ML allows systems to learn from data without being explicitly programmed.
Deep Learning, a subset of ML, uses artificial neural networks with multiple layers to model and process complex patterns in data. These neural networks are inspired by the structure and function of the human brain.
B. Advantages in the Pharmaceutical Industry
The pharmaceutical industry is awash with data – from genomic sequences and protein structures to clinical trial results and patient records. This wealth of information presents both a challenge and an opportunity. While it’s impossible for human researchers to process and analyze all this data manually, AI and ML thrive on large datasets.
Here are some key advantages of AI and ML in the pharmaceutical industry:
- Data Processing: AI can analyze vast amounts of biomedical data at speeds far beyond human capability.
- Pattern Recognition: ML algorithms can identify complex patterns and relationships in data that might be missed by human researchers.
- Prediction: AI models can make predictions about drug-target interactions, potential side effects, and drug efficacy based on existing data.
- Cost Reduction: By streamlining various stages of the drug discovery process, AI can potentially reduce the overall cost of drug development.
- Time Efficiency: AI-driven approaches can significantly accelerate the drug discovery and repurposing process.
- Personalized Medicine: AI can help identify patient subgroups most likely to respond to specific treatments, paving the way for more personalized therapeutic approaches.
As Dr. Atul Butte, a pioneer in biomedical informatics at the University of California, San Francisco, puts it:
“There’s a lot of data out there, and we can use it to drive the discovery of new drugs. We have more information about biology and medicine than ever before. The problem is, we can’t read it all. But AI can.”
With these advantages in mind, let’s explore how AI and ML are specifically being applied to drug repurposing.
IV. AI and ML Techniques in Drug Repurposing
A. Machine Learning Algorithms
Machine Learning algorithms form the backbone of AI-driven drug repurposing efforts. These algorithms can be broadly categorized into supervised, unsupervised, and semi-supervised learning methods.
- Supervised Learning: These algorithms learn from labeled data to make predictions. In drug repurposing, they might be used to predict drug-target interactions or drug efficacy based on known examples. Example: Support Vector Machines (SVMs) have been used to predict new indications for existing drugs based on their chemical structures and known targets.
- Unsupervised Learning: These algorithms find patterns in unlabeled data. They’re particularly useful for discovering hidden relationships in complex biological datasets. Example: Clustering algorithms can group drugs with similar mechanisms of action, potentially revealing new therapeutic applications.
- Semi-supervised Learning: These methods use both labeled and unlabeled data, which can be particularly useful when labeled data is scarce – a common scenario in drug repurposing. Example: Graph-based semi-supervised learning has been used to predict drug-disease associations by integrating multiple types of biological data.
B. Deep Learning Models
Deep Learning, a subset of ML, has shown remarkable promise in drug repurposing due to its ability to handle complex, high-dimensional data.
- Convolutional Neural Networks (CNNs): Originally developed for image recognition, CNNs have been adapted for tasks like predicting drug-target interactions based on 3D structural data.
- Recurrent Neural Networks (RNNs): These are particularly useful for analyzing sequential data, such as genomic sequences or time-series clinical data.
- Graph Neural Networks (GNNs): These models can capture complex relationships in graph-structured data, making them ideal for analyzing biological networks and predicting drug-target interactions.
“Ultimately, the synergy between AI and drug repurposing has therefore been defined as holding promises for addressing the unmet medical needs in rare diseases.”[4]
C. Natural Language Processing
Natural Language Processing (NLP) is a branch of AI that deals with the interaction between computers and human language. In drug repurposing, NLP plays a crucial role in extracting valuable information from unstructured text data, such as scientific literature, clinical trial reports, and electronic health records.
- Text Mining: NLP algorithms can automatically extract relevant information about drugs, diseases, and their relationships from vast amounts of scientific literature.
- Named Entity Recognition: This technique identifies and classifies named entities in text into predefined categories like drugs, genes, proteins, and diseases.
- Relationship Extraction: NLP can identify relationships between entities, such as drug-drug interactions or drug-disease associations.
- Topic Modeling: This technique can identify underlying themes in large collections of documents, potentially revealing new connections between drugs and diseases.
Dr. Ying Ding, a professor of informatics at the University of Texas at Austin, emphasizes the importance of NLP in drug repurposing:
“Natural Language Processing allows us to tap into the collective knowledge of the scientific community. By analyzing millions of research papers, we can uncover hidden connections and generate new hypotheses for drug repurposing.”
These AI and ML techniques, working in concert, are revolutionizing the way we approach drug repurposing. But to truly understand their impact, we need to look at the data sources they’re working with.
V. Data Sources for AI-Driven Drug Repurposing
The power of AI in drug repurposing lies not just in its algorithms, but in the vast and diverse data sources it can analyze. Let’s explore some of the key data types that fuel AI-driven drug repurposing efforts.
A. Genomic Data
Genomic data provides crucial insights into the genetic basis of diseases and drug responses. AI algorithms can analyze this data to identify potential drug targets and predict drug efficacy.
Key sources of genomic data include:
- Genome-Wide Association Studies (GWAS): These studies identify genetic variants associated with specific diseases.
- Gene Expression Data: This data reveals how genes are expressed under different conditions, including in response to drugs.
- Epigenomic Data: Information about chemical modifications to DNA that affect gene expression without changing the DNA sequence.
B. Chemical Structures
The chemical structure of drugs is a crucial factor in determining their biological activity. AI models can analyze structural data to predict:
- Drug-target interactions
- Potential side effects
- New therapeutic applications
Sources of chemical structure data include:
- PubChem: A database of chemical molecules and their activities against biological assays.
- ChEMBL: A manually curated database of bioactive molecules with drug-like properties.
C. Clinical Trial Data
Clinical trial data provides valuable information about drug efficacy and safety in human subjects. AI can analyze this data to:
- Identify potential new indications for existing drugs
- Predict drug responses in different patient populations
- Optimize clinical trial design for repurposed drugs
Key sources include:
- ClinicalTrials.gov: A database of privately and publicly funded clinical studies conducted around the world.
- FDA Adverse Event Reporting System (FAERS): A database of adverse event reports submitted to the FDA.
D. Scientific Literature
The vast corpus of scientific literature contains a wealth of information about drugs, diseases, and biological mechanisms. NLP techniques can extract valuable insights from this unstructured data.
Sources include:
- PubMed: A database of biomedical literature.
- MEDLINE: A bibliographic database of life sciences and biomedical information.
Dr. Russ Altman, a professor of bioengineering at Stanford University, highlights the importance of integrating diverse data sources:
“The key to successful AI-driven drug repurposing is the ability to integrate and analyze diverse types of data. By combining genomic, chemical, clinical, and literature data, we can gain a more comprehensive understanding of drug-disease relationships and identify promising repurposing opportunities.”
Now that we’ve explored the data sources, let’s dive into the key applications of AI in drug repurposing.
VI. Key Applications of AI in Drug Repurposing
AI and ML are being applied to various aspects of the drug repurposing process. Here are some of the key applications:
A. Target Identification
One of the primary applications of AI in drug repurposing is identifying new drug targets. AI algorithms can analyze genomic and proteomic data to:
- Identify genes or proteins associated with specific diseases
- Predict the druggability of potential targets
- Discover novel biological pathways that could be targeted by existing drugs
For example, researchers at the University of Cambridge used a ML approach to identify a new target for treating rheumatoid arthritis. Their algorithm analyzed gene expression data and predicted that inhibiting a specific enzyme could reduce inflammation in joint tissue.
B. Drug-Target Interaction Prediction
Once potential targets are identified, AI can predict how existing drugs might interact with these targets. This involves:
- Analyzing the chemical structures of drugs
- Predicting binding affinities between drugs and targets
- Simulating drug-target interactions at the molecular level
A team at MIT used deep learning to predict drug-target interactions across multiple cancer types. Their model, trained on a large dataset of known interactions, was able to identify several promising drug-target pairs for further investigation.
C. Side Effect Prediction
Predicting potential side effects is crucial in drug repurposing. AI models can:
- Analyze chemical structures to predict toxicity
- Identify potential drug-drug interactions
- Predict adverse events based on clinical trial data and post-marketing surveillance
For instance, researchers at Stanford University developed a deep learning model that can predict potential side effects of drug combinations. This could be particularly valuable in repurposing drugs for complex diseases that require combination therapies.
D. Drug Combination Prediction
Many diseases, particularly complex ones like cancer, often require combination therapies. AI can help identify potentially effective drug combinations by:
- Analyzing synergistic effects between drugs
- Predicting the efficacy of drug combinations in specific patient populations
- Optimizing dosing regimens for drug combinations
A study published in Nature Communications used a ML approach to predict synergistic drug combinations for cancer treatment. The algorithm analyzed data on drug sensitivity and gene expression to identify combinations
Citations:
[1] https://www.ncbi.nlm.nih.gov/pmc/articles/PMC8783773/
[2] https://drugrepocentral.scienceopen.com/hosted-document?doi=10.58647%2FDRUGARXIV.PR000007.v1
[3] https://www.frontiersin.org/journals/medicine/articles/10.3389/fmed.2024.1404338/full
[4] https://www.ncbi.nlm.nih.gov/pmc/articles/PMC11150798/
[5] https://www.nature.com/articles/s41467-021-21330-0
[6] https://www.sciencedirect.com/science/article/pii/S2590257121000298
[7] https://arpa-h.gov/news-and-events/arpa-h-awards-ai-driven-project-repurpose-approved-medications
[8] https://drugrepocentral.scienceopen.com/hosted-document?doi=10.58647%2FDRUGREPO.24.1.0004